Not too long ago, misguided pundits predicted that almost all enterprise workloads would eventually migrate to the public cloud. They mentioned benefits like scalability, performance and cost as major contributing factors to an impending public cloud revolution. They were right about many of the motivating factors, but missed the mark on exactly how and where those benefits would be best achieved—rather than public cloud, hybrid cloud has quickly become the de facto infrastructure for today’s leading organizations.
So, while nearly every company will use the public cloud for certain workloads, they will also operate on-premises data center (DC) infrastructure for others. With this reality established, the traditional, on-premises data center is now undeniably roaring back into the spotlight. And with artificial intelligence (AI) in the mix, the industry has seemingly moved on with every strategist and technologist now trying to figure out how to best use data center infrastructure to execute new AI projects. At Juniper Networks, we have the answer, with an AI data center solution that offers 55% TCO savings versus alternatives, with comparable performance.
AI Data Centers Are Built Differently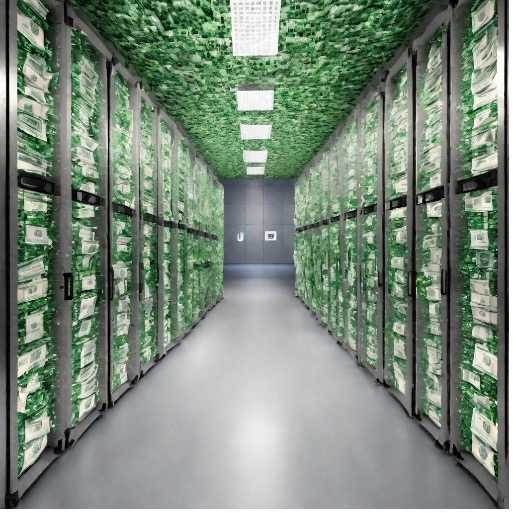
The first thing to understand is that DC infrastructure optimized for AI workloads is built differently, especially when those data centers are specifically designed to train AI applications such as large language models (LLMs). AI workloads exhibit different traffic patterns and flow characteristics, which require innovative new data center network architecture designs and protocols.
Thousands of technology experts at Juniper have devoted their careers to solving tricky challenges like this. But we’re also intensely curious about how the economics of building and operating these new AI data centers may financially impact our customers, so we engaged ACG Research to help build an objective viewpoint on the following questions:
- How are the overall economics of traditional data centers different from AI training data centers?
- Is it more economical to use Ethernet or InfiniBand for AI training data center networking?
- Is it more economical to build your own private data center for AI or should you use a public cloud provider?
ACG conducted extensive financial modelling to dig into these questions. Let’s take a quick look at what they found.
Traditional Data Center vs. AI Training Data Center
Excluding real estate and power facilities, data center infrastructure essentially consists of three components: compute, storage and networking. Traditional data centers use ubiquitous central processing units (CPUs) as the primary compute engine, while AI training data centers use expensive graphics processing units (GPUs). A GPU server with 8 GPUs typically costs hundreds of thousands of dollars – this is more than 10 times the cost of a standard, CPU-based server. As shown in the following table, compute dominates the cost of an AI training data center, while the networking portion is very small:
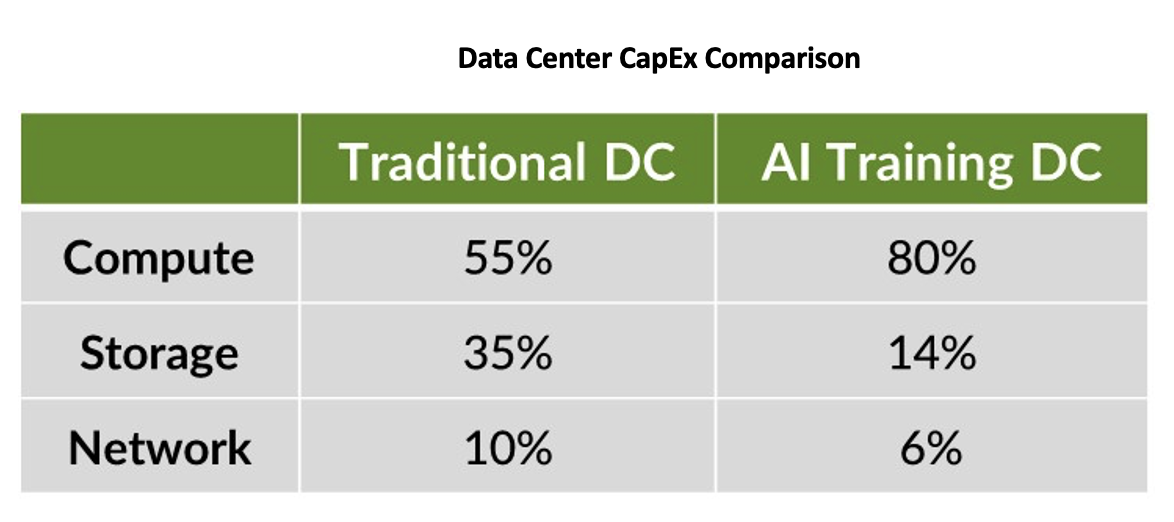
However, it would be a mistake to conclude that the network is less important for AI training. In fact, it’s quite the opposite. Optimizing GPU efficiency is the key to containing overall AI costs, and the network plays a critical role in this objective. AI training is a massive parallel processing problem. Job completion time (JCT) is the key metric. When done right, all GPUs work together efficiently and effectively. Do it wrong and you unnecessarily waste a lot of time and money, and nobody wants that.
How It Works
To train an AI model, massive data sets are chopped into smaller chunks and distributed as flows across a backend DC fabric. This goes to dispersed GPUs that perform calculations, and then the network merges those individual results together before another training run begins. Any straggler GPU computations cause delays, or “tail latency.” As this training process repeats, sometimes for months, these issues compound and can add up to significant hold-ups. This is where the network comes into the story. For a fraction of the cost of the overall AI data center, you can ensure that the right network is helping you optimize overall ROI. In other words, spend a little more time and money to design the network up front and you’ll save a lot more on the overall time and cost to deploy the AI application in the end.
Juniper AI-Optimized Ethernet Fabric vs. NVIDIA InfiniBand for AI Data Center Networks
The InfiniBand networking technology emerged in 1999 as a niche solution to handle the special demands of high-performance compute (HPC) applications. Because of this history, InfiniBand currently dominates AI training data centers, but the Ethernet standard is rapidly gaining share. To compare the costs of an AI data center with an NVIDIA InfiniBand networking solution versus the Juniper AI-Optimized Ethernet solution, ACG built a financial model – and the results are intriguing.

As indicated in the table above, the Juniper solution provides a 55% lower total cost of ownership (TCO) versus NVIDIA InfiniBand. The initial CapEx outlay, which consists of switches, cables, optics and software, is much higher for InfiniBand since it is effectively single-sourced. ACG estimates additional, substantial OpEx savings from Juniper, due primarily to the proven benefits of the Juniper Apstra data center fabric management and automation software and the limited availability of open, vendor-neutral automation platforms available for InfiniBand. Accounted for as an operational expense in the ACG model, Apstra demonstrates the conclusive advantages of an open Ethernet ecosystem versus a closed system solution.
Although InfiniBand is a strong technical solution, there are several additional advantages of Ethernet. Ethernet networks are proven, ubiquitous and well understood, while far fewer engineers are familiar with the intricacies of InfiniBand. Another point to consider is that the vast Ethernet vendor ecosystem will continue to push down costs and drive innovation much faster than InfiniBand. Furthermore, this analysis is limited to a single AI training backend data center, while the remainder of an enterprise’s data center infrastructure is almost always Ethernet, including even frontend AI data centers typically used for inference. Common NetOps across frontend and backend AI data centers is another substantial advantage of Ethernet, as well as Juniper Apstra in particular.
In short, Ethernet will continue to become better, faster and cheaper for networking AI data center infrastructure. Keep in mind that the ACG analysis above doesn’t include these expected additional benefits.
But what about performance? A comparison of Ethernet versus InfiniBand solely based on economics is not sufficient. Ethernet networking solutions must also demonstrate at least comparable performance to InfiniBand. Broadcom finds that Ethernet improves AI job completion time by 10% and provides 30x faster failover vs. InfiniBand. In addition, Juniper has built an AI lab at our headquarters in Sunnyvale to benchmark Ethernet performance as well as test Juniper Validated Designs (JVDs). We tune data center fabrics to ensure optimal congestion management and load balancing and ease the burden of our customers having to do this. We have shown many customers that Ethernet performance beats InfiniBand for many use cases. Recently, Juniper demonstrated an industry first, benchmarking an LLM on a multi-node AI inference fabric with MLCommons. And look for more benchmark testing and additional collaboration between Juniper and MLCommons in the future.
Private Data Center vs. Public Cloud for AI
Five or ten years ago, enterprises started rushing to public cloud, enticed by utopian promises of greater flexibility and lower cost. However, most companies quickly realized that public cloud is not as simple and cheap as so many had thought. “Cloud regret” is the new standard as we hear countless anecdotes of companies repatriating workloads back to private, on-premises data centers. With respect to AI data center infrastructure plans, we now see companies wrestling with these same decisions, so we asked ACG to take a deeper look at the economics of running AI workloads on private data center versus public cloud.
ACG compared the cost of running AI workloads with Amazon Web Services (AWS) versus on-premises private infrastructure. The AWS solution consists of GPUs (p5.48xlarge EC2 instances), FSX cluster high-performance storage and egress expenses. To accurately compare the AWS cloud to an on-premises solution, ACG modelled the private data center solution with Juniper networking from the previous scenario with the following additions: rack & stack, cabinets, professional services, power and cooling and facilities expenses. The important financial difference is that the AWS solution is all OpEx with no CapEx, while the Juniper on-premises solution has both OpEx and CapEx components.
ACG found that over a three-year period, the Juniper private data center solution offers a 46% lower TCO.
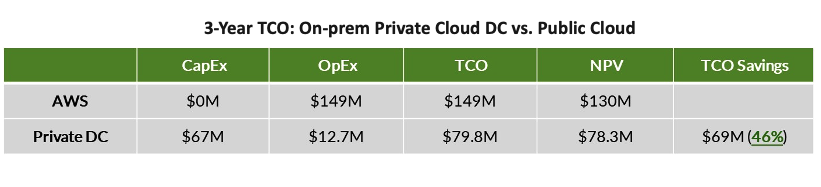
To Rent or to Build, That is the Question
Building out a new GPU cluster is expensive, but so is renting that capacity from a hyperscaler. On the heels of the private versus public cloud debate, we expect companies will continue to get smarter about looking at private versus public cloud for AI. With runtime under our collective belts, the decisions around “build” (enterprise on-premises or in a co-location facility) versus “rent” (public cloud) versus “hybrid” (both) will be made more thoughtfully. Beyond narrow cost considerations, many companies will opt to build on-premises AI data centers for drivers like better control or improved security, or they’ll make those decisions based on issues related to intellectual property, compliance and regulations. Tom Nolle of Andover Intel recently remarked that “my data from enterprises says that the total usage of AI by enterprises would be at least three times greater if they were able to secure their data on premises.”
Learn More About What Juniper Can Do for You
Gain more valuable insights into the economics of AI data centers and see more details of the financial analysis by reading the ACG Research report here. And be sure to attend “Seize the AI Moment”, our online event, on July 23rd where we have an all-star lineup of industry luminaries to talk about what they’ve learned in serving this burgeoning market.